Vision-language refers to the field of artificial intelligence that aims to develop systems capable of understanding and generating both visual and textual information. The goal is to enable machines to perceive the world as humans do, by combining the power of computer vision with natural language processing.
Understanding the Basics: Vision and Language
At its core, vision-language is about bridging the gap between two distinct modalities: vision and language. Vision involves the ability to perceive and interpret visual information, such as images and videos, while language is the system of communication that humans use to convey meaning through words and sentences.
By combining these two modalities, vision-language systems can enable machines to understand the visual world and communicate about it in a more natural and human-like way.
Image Captioning
This has numerous applications, from enhancing human-machine communication to improving image and video search capabilities. One area where vision-language is making significant progress is in image captioning, where machines are trained to generate textual descriptions of images.
This involves developing deep learning models that can analyse an image and generate a corresponding natural language description. This can be especially useful for individuals with visual impairments or for search engines looking to better understand the content of images.
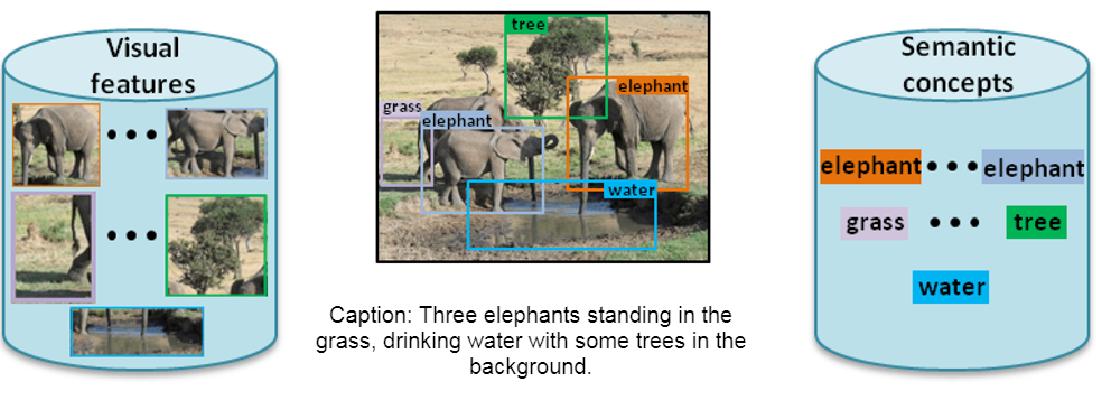
Visual Question Answering
Another application of vision-language is in visual question answering (VQA), where machines are trained to answer questions based on visual information. This involves combining computer vision and natural language processing to enable machines to understand both the visual information and the meaning behind the questions being asked.
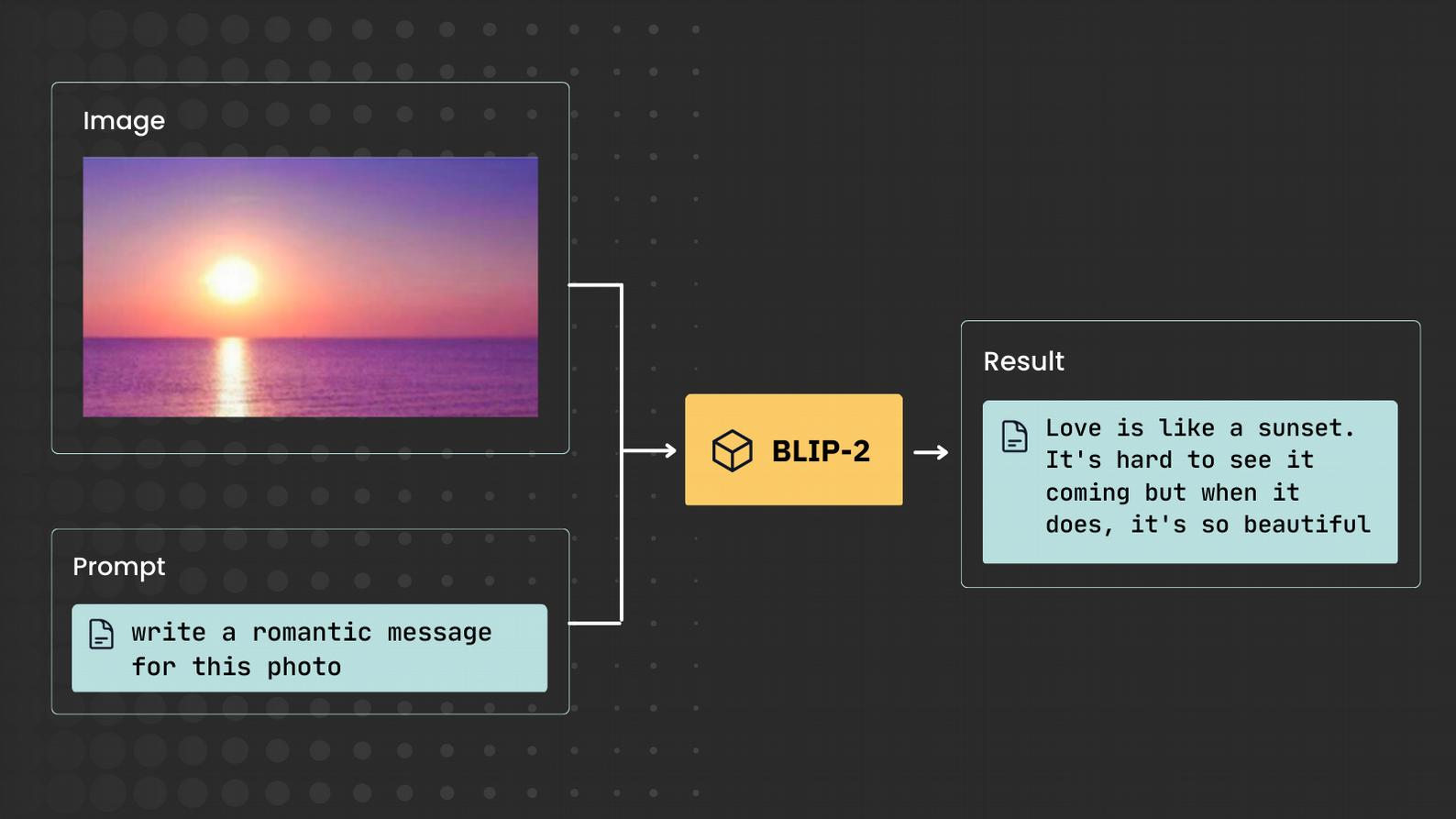
One major challenge in the field of vision-language is developing systems that can understand the nuances of language and context. For example, understanding the difference between “a red car” and “a car that is red” requires a deep understanding of language and context that is difficult to replicate in machines.
Despite these challenges, vision-language is a rapidly growing field with tremendous potential to revolutionise how machines interact with and understand the visual world.
Storytelling
As technology continues to advance, we can expect to see even more exciting applications of vision-language in the years to come. Another area where vision-language is making strides is in visual storytelling, where machines are trained to generate a narrative or a story from a sequence of images or videos. This involves developing models that can understand the visual content and generate a coherent and engaging story that is both natural and human-like.
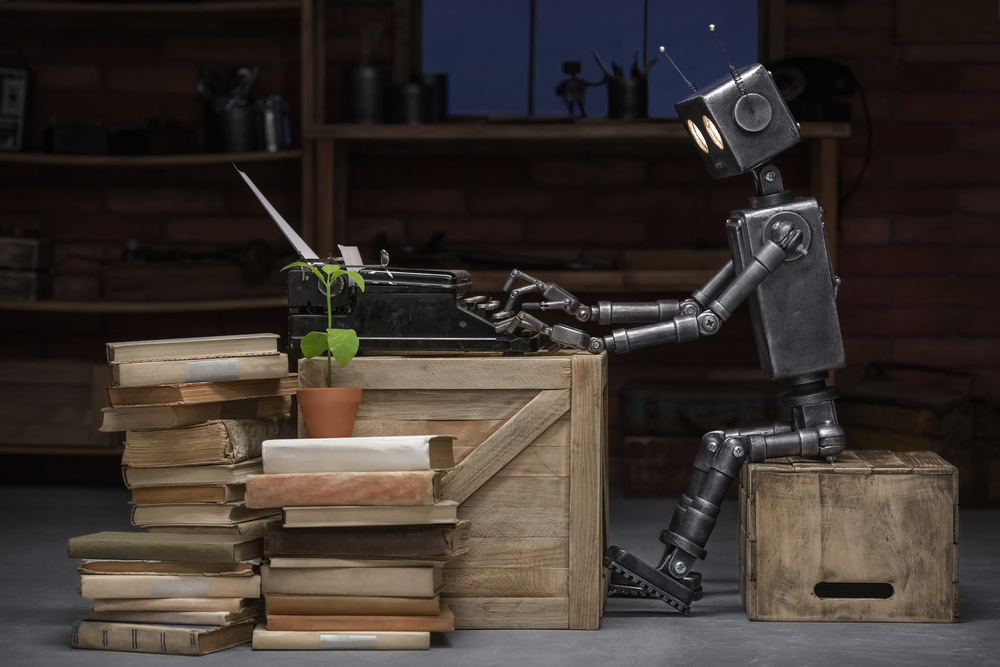
Vision-language also has implications in the fields of education and healthcare. For instance, machines can be trained to understand medical images and provide more accurate diagnoses. In the education sector, vision-language can be used to develop more interactive and engaging learning materials that combine visual and textual information.
One exciting development in the field of vision-language is the use of pre-trained language models such as GPT and BERT to improve the performance of computer vision models. By combining pre-trained language models with computer vision models, machines can be trained to perform more complex tasks such as image retrieval, image synthesis, and image recognition with greater accuracy and efficiency.
However, as with any emerging technology, there are also ethical considerations to be aware of in the field of vision-language. For instance, the use of vision-language in surveillance systems raises concerns about privacy and individual rights.
It is important for researchers and practitioners in the field to consider the ethical implications of their work and develop systems that are designed with fairness, transparency, and accountability in mind.
One of the main drivers of progress in the field of vision-language is the availability of large-scale datasets. These datasets contain millions of images and corresponding textual descriptions or annotations, and they are used to train and evaluate vision- language models. Popular datasets in the field include COCO (Common Objects in Context), Visual Genome, and Flickr30k.
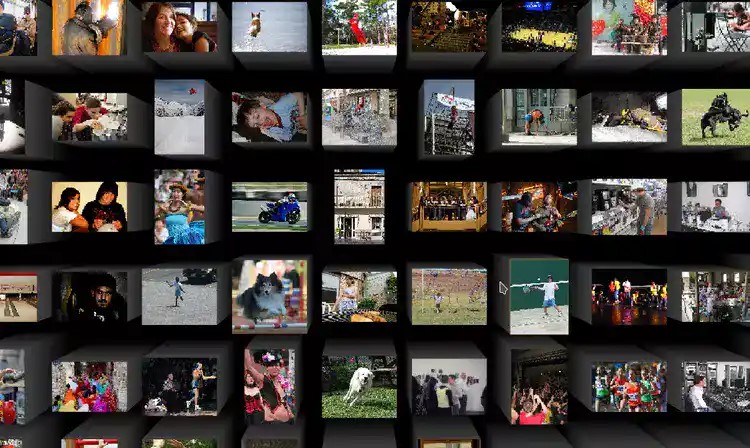
In addition to datasets, the field of vision-language is also supported by a variety of tools and frameworks. These include deep learning libraries such as TensorFlow and PyTorch, as well as specialised vision-language libraries such as MMF (Multimodal Framework) and Hugging Face, Transformers.
Another important aspect of vision- language research is the evaluation of models. Because vision-language models can be used for a variety of tasks, it is important to have standardised evaluation metrics that can measure performance across different domains. Popular metrics include
BLEU (Bilingual Evaluation Understudy), ROUGE (Recall-Oriented Understudy for Gisting Evaluation), and METEOR (Metric for Evaluation of Translation with Explicit ORdering).
Finally, it is worth noting that vision-language is a highly interdisciplinary field, with researchers and practitioners from computer science, linguistics, psychology, and other disciplines contributing to its development. This cross-disciplinary collaboration has been critical to the progress of the field and will continue to be important in the future.
In conclusion, vision-language is an exciting and rapidly evolving field that has the potential to transform how machines interact with and understand the visual world. With the availability of large-scale datasets, powerful deep learning libraries, and standardised evaluation metrics, we can expect to see continued progress in the development of vision-language models and applications in a range of domains.
In VOXReality, our mission is to develop vision-language models that will be useful in a variety of applications, such as assisting people or improving the accessibility of digital content. Specifically, our tooling will generate captions or summaries for videos, which could benefit content creators, journalists or educators.
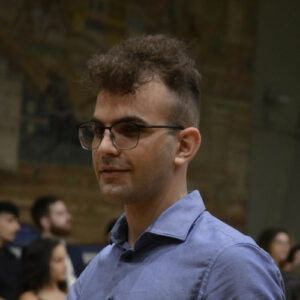
Stefanos Biliousis
Hello! My name is Stefanos Biliousis and I'm a computer vision researcher with a passion for exploring the latest advances in artificial intelligence. With a background in machine learning and computer science, I spend my days developing innovative algorithms and techniques for image and video analysis. I'm fascinated by the many ways that computer vision and AI are revolutionizing the world around us.